prof. dr. sc. Damir Seršić
project leader
full professor, Department of Electronic Systems and Information Processing
e-mail: damir.sersic@fer.hr
|
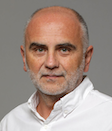 |
prof. dr. sc. Davor Petrinović
full professor, Department of Electronic Systems and Information Processing
e-mail: davor.petrinovic@fer.hr
|
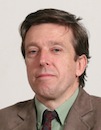 |
izv. prof. dr. sc. Ana Sović Kržić
associate professor, Department of Electronic Systems and Information Processing
e-mail: ana.sovic@fer.hr
|
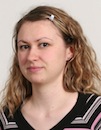 |
izv. prof. dr. sc. Azra Tafro
associate professor, University of Zagreb Faculty of Forestry
e-mail: azra.tafro@fer.hr
|
 |
dr. sc. Ivan Ralašić
external associate, CTO of Forsight
e-mail: ivan.ralasic@fer.hr
|
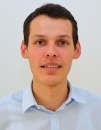 |
dr. sc. Tin Vlašić
external associate, Postdoctoral Research Fellow at A*STAR Singapore
e-mail: tin.vlasic@fer.hr
|
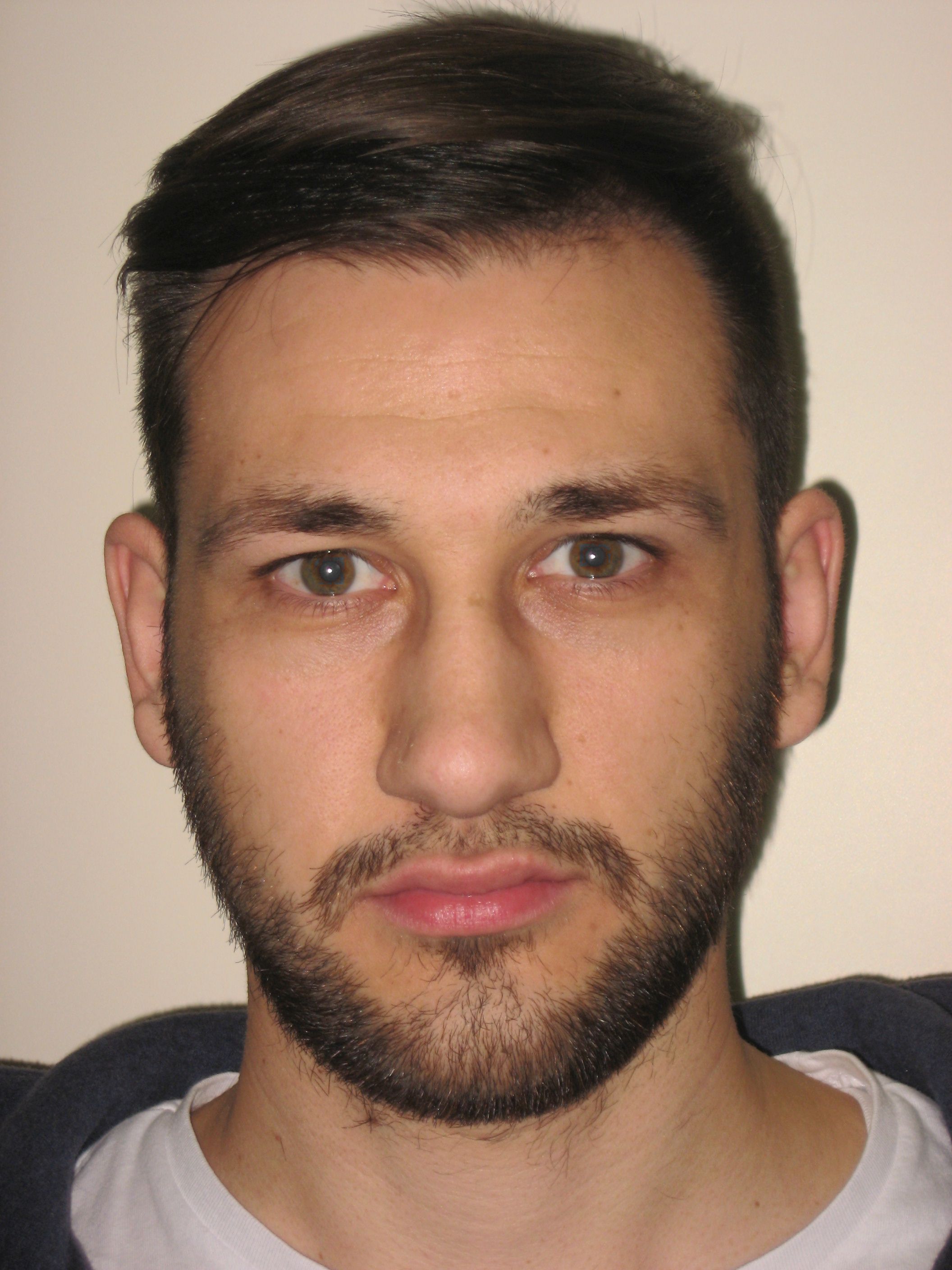 |
Tomislav Matulić, mag. ing.
assistant, Department of Electronic Systems and Information Processing
e-mail: tomislav.matulic@fer.hr
|
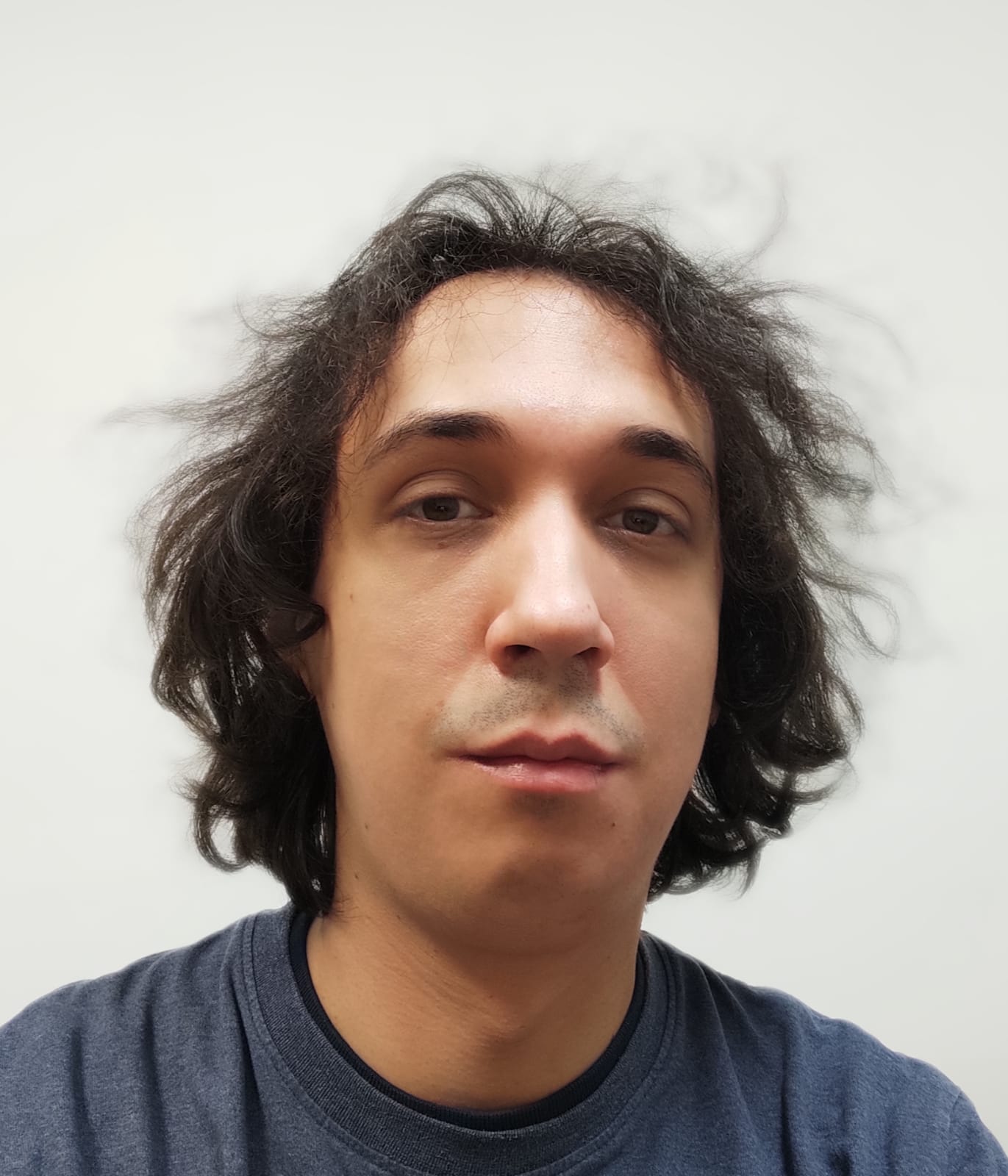 |
Ivan Bukić, mag. ing.
assistant, Department of Electronic Systems and Information Processing
e-mail: ivan.bukic@fer.hr
|
|
Journal articles
T. Matulić and D. Seršić, "Stochastic model for enhanced PET image reconstruction", Biomedical Signal Processing and Control, vol. 94, no. 106294, 2024. doi: 10.1016/j.bspc.2024.106294 |
A. Tafro and D. Seršić, "Gaussian Mixture Estimation from Lower-Dimensional Data with Application to PET Imaging", Mathematics, vol. 12 (5), no. 764, 2024. doi: 10.3390/math12050764 |
A. Gribl Koščević and D. Petrinović, "A Fast Method for Fitting a Multidimensional Gaussian Function," in IEEE Access, vol. 10, pp. 106921-106935, 2022. doi: 10.1109/ACCESS.2022.3212388 |
A. Tafro, D. Seršić and A. Sović Kržić, "2D PET Image Reconstruction Using Robust L1 Estimation of the Gaussian Mixture Model," Informatica, vol. 33, no. 3, pp. 653–669, 2022. doi: 10.15388/22-INFOR482 |
T. Vlašić and D. Seršić, "Single-pixel Compressive Imaging in Shift-Invariant Spaces via Exact Wavelet Frames," Signal Processing: Image Communication, vol. 105, article no. 116702, July 2022. doi: 10.1016/j.image.2022.116702 |
T. Vlašić and D. Seršić, "Sampling and Reconstruction of Sparse Signals in Shift-Invariant Spaces: Generalized Shannon's Theorem Meets Compressive Sensing," IEEE Transactions on Signal Processing, vol. 70, pp. 438-451, 2022. doi: 10.1109/TSP.2022.3141009 |
A. Gribl and D. Petrinović, "A Robust Method for Gaussian Profile Estimation in the Case of Overlapping Objects," IEEE Access, vol. 9, pp. 21071-21084, 2021. doi: 10.1109/ACCESS.2021.3055282 |
I. Ralašić, D. Seršić and S. Šegvić, "Perceptual Autoencoder for Compressive Sensing Image Reconstruction," Informatica, vol. 31, no. 3, pp. 561-578, 2020. doi: 10.15388/20-INFOR421
|
I. Ralašić, M. Đonlić and D. Seršić, "Dual Imaging–Can Virtual Be Better Than Real?," IEEE Access, vol. 8, pp. 40246-40260, 2020. doi: 10.1109/access.2020.2976870
|
N. Dlab, F. Cimermančić, I. Ralašić and D. Seršić, "Overcoming spatio-angular trade-off in light field acquisition using compressive sensing," Automatika, vol. 61, no. 2, pp. 250-259, 2020. doi: 10.1080/00051144.2020.1715582
|
T. Vlašić, I. Ralašić, A. Tafro and D. Seršić, "Spline-like Chebyshev polynomial model for compressive imaging," Journal of Visual Communication and Image Representation, vol. 66, article 102731, 2020. doi: 10.1016/j.jvcir.2019.102731
|
Conference papers
T. Vlašić, T. Matulić and D. Seršić, "Estimating Uncertainty in PET Image Reconstruction via Deep Posterior Sampling", Proceedings of Machine Learning Research, Medical Imaging with Deep Learning (MIDL2023), Nashville (TN), USA, 2023. link |
D. Potoč and D. Petrinović, "Estimating a nonradial vignetting shape", 2023 46th MIPRO ICT and Electronics Convention (MIPRO), Opatija, Croatia, 2023. doi: 10.23919/MIPRO57284.2023.10159695 |
T. Vlašić, H. Nguyen, A. Khorashadizad and I. Dokmanić, "Implicit Neural Representation for Mesh-Free Inverse Obstacle Scattering", 2022 56th Asilomar Conference on Signals, Systems, and Computers, Pacific Grove (CA), USA, 2022. doi: 10.1109/IEEECONF56349.2022.10052055 |
A. Gribl Koščević and D. Petrinović, "Maximizing Accuracy of 2D Gaussian Profile Estimation Using Differential Entropy," 2022 45th Jubilee International Convention on Information, Communication and Electronic Technology (MIPRO), Opatija, Croatia, 2022, pp. 888-893, doi: 10.23919/MIPRO55190.2022.9803382 |
D. Potoč and D. Petrinović, "Creating a synthetic image for evaluation of vignetting modeling and estimation", 2022 45th Jubilee International Convention on Information, Communication and Electronic Technology (MIPRO), Opatija, Croatia, 2022, pp. 918-923, doi: 10.23919/MIPRO55190.2022.9803709 |
A. Gribl and D. Petrinović, "The Influence of Noise on 2D Gaussian Profile Parameters Estimation," 2021 44th International Convention on Information, Communication and Electronic Technology (MIPRO), Opatija, Croatia, 2021, pp. 1032-1037, doi: 10.23919/MIPRO52101.2021.9596661 |
T. Matulić, R. Bagarić and D. Seršić, "Enhanced reconstruction for PET scanner with a narrow field of view using backprojection method," 2021 44th International Convention on Information, Communication and Electronic Technology (MIPRO), Opatija, Croatia, 2021, pp. 317-322, doi: 10.23919/MIPRO52101.2021.9596922 |
T. Vlašić and D. Seršić, "Multilevel Subsampling of Principal Component Projections for Adaptive Compressive Sensing," 2021 12th International Symposium on Image and Signal Processing and Analysis (ISPA), Zagreb, Croatia, 2021, pp. 29-35, doi: 10.1109/ISPA52656.2021.9552127 |
A. Gribl Koščević and D. Petrinović, "Extra-low-dose 2D PET imaging," 2021 12th International Symposium on Image and Signal Processing and Analysis (ISPA), Zagreb, Croatia, 2021, pp. 84-90, doi: 10.1109/ISPA52656.2021.9552059 |
T. Vlašić and D. Seršić, "Sub-Nyquist Sampling in Shift-Invariant Spaces," 2020 28th European Signal Processing Conference (EUSIPCO), Amsterdam, Netherlands, 2021, pp. 2284-2288, doi: 10.23919/Eusipco47968.2020.9287712
|
I. Ralašić, M. Đonlić and D. Seršić, "High-Resolution View Synthesis In Camera-Projector Systems Using Compressive Dual Imaging," 2020 3rd International Conference on Sensors, Signal and Image Processing (SSIP), Prague, Czech Republic, 2020, pp. 7-12, doi: 10.1145/3441233.3441236
|
K. Sever, T. Vlašić and D. Seršić, "A Realization of Adaptive Compressive Sensing System," 2020 43rd International Convention on Information, Communication and Electronic Technology (MIPRO), Opatija, Croatia, 2020, pp. 152-157, doi: 10.23919/MIPRO48935.2020.9245389
|
A. Gribl and D. Petrinović, "Synthetic astronomical image sequence generation," 2020 43rd International Convention on Information, Communication and Electronic Technology (MIPRO), Opatija, Croatia, 2020, pp. 1103-1108, doi: 10.23919/MIPRO48935.2020.9245294
|
Other
A. Tafro, "Gaussian mixture model for PET image reconstruction with reduced sample size", 26th International Scientific Symposium on Biometrics (BIOSTAT2023), Zadar, Croatia, 2023. |
T. Matulić and D. Seršić, "Probabilistic description of rotational 3D PET systems", 8th Int'l Workshop on Data Science (IWDS 2023), Zagreb, Croatia, 2023. |
D. Potoč and D. Petrinović, "Estimation of non-radial vignetting using minimization procedures," Abstract Book of the 7th International Workshop on Data Science (IWDS), Zagreb, Croatia, pp. 17-19, 2022. |
A. Gribl Koščević and D. Petrinović, "Fitting an elliptical paraboloid with the known shape to the empirical data", 7th International Workshop on Data Science (IWDS 2022), Zagreb, Croatia, 2022. |
A. Gribl Koščević and D. Petrinović, "Fitting an elliptical paraboloid with the known shape to the empirical data," Abstract Book of the 7th International Workshop on Data Science (IWDS), Zagreb, Croatia, pp. 13-15, 2022. |
T. Matulić, R. Bagarić and D. Seršić, "Fast reconstruction for PET scanner with incomplete sector set," Abstract Book of the 6th International Workshop on Data Science (IWDS), Zagreb, Croatia, pp. 19-21, 2021. |
T. Vlašić and D. Seršić, "Statistical Compressive Sensing of Analog Signals in B-Spline Function Spaces," Abstract Book of the 5th International Workshop on Data Science (IWDS), Zagreb, Croatia, pp. 28-31, 2020.
|
A. Gribl and D. Petrinović, "The influence of the Huber estimator tuning constant on the performance of the iteratively reweighted least squares method," Abstract Book of the 5th International Workshop on Data Science (IWDS), Zagreb, Croatia, pp. 12-14, 2020.
|
|